AI Integration in Business: Benefits, Strategies, & Real-World Applications
In today’s fast-paced digital economy, artificial intelligence (AI) integration is no longer just a tech trend—it’s a critical enabler of business transformation. Companies across industries are embedding AI into their core systems to operate smarter, faster, and more competitively.
But what exactly does AI integration involve? How can organizations overcome technical and operational challenges to deploy AI effectively? And what kind of real-world impact can they expect? Dive into our blog to find out the answers.
Table of Contents
- What Is AI Integration?
- How Does AI Integration Work?
- Benefits of AI Integration for Businesses
- Real-World Applications of AI Integration (with Case Studies)
- Top Challenges of AI Integration
- AI Integration Strategies for Long-Term Success
- The Future of AI Integration
- How Kaopiz Helps Businesses with AI Integration
- Conclusion
- FAQs
What Is AI Integration?
AI integration is the process of embedding artificial intelligence technologies, such as machine learning (ML), natural language processing (NLP), and predictive analytics, into existing systems, applications, and workflows. The goal is to enhance functionality, improve efficiency, and enable faster, smarter decision-making.
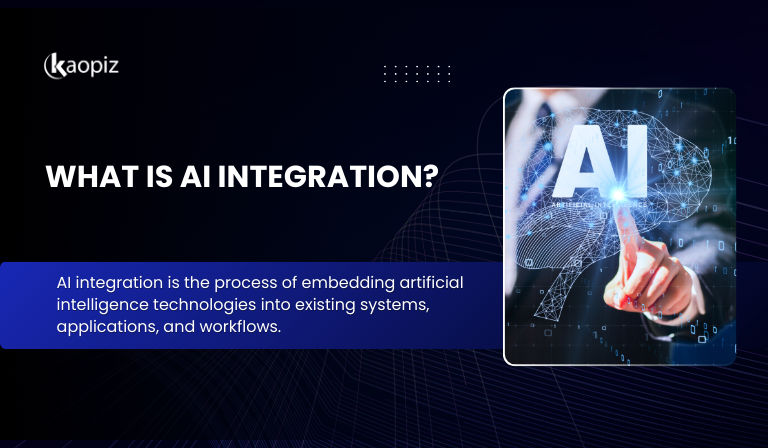
For example:
By analyzing data, learning patterns, and continuously improving, integrated AI can automate routine tasks, deliver intelligent recommendations, and surface insights that were previously difficult or time-consuming to obtain.
How Does AI Integration Work?
AI integration works by embedding algorithms or AI models into an organization’s digital infrastructure. These models process data in real time or in batches and use techniques like machine learning and deep learning to uncover trends, predict outcomes, and optimize processes.
Whether through cloud APIs, on-premises deployment, or embedded tools, AI becomes part of the operational backbone, empowering businesses to innovate and scale intelligently.
Benefits of AI Integration for Businesses
Integrating AI into business systems delivers measurable value across departments—from streamlining workflows to enhancing customer engagement and uncovering new growth opportunities.
According to a 2023 McKinsey report, businesses using AI-driven decision-making saw a 20% increase in revenue and a 30% reduction in operational costs compared to those relying solely on traditional analytics.
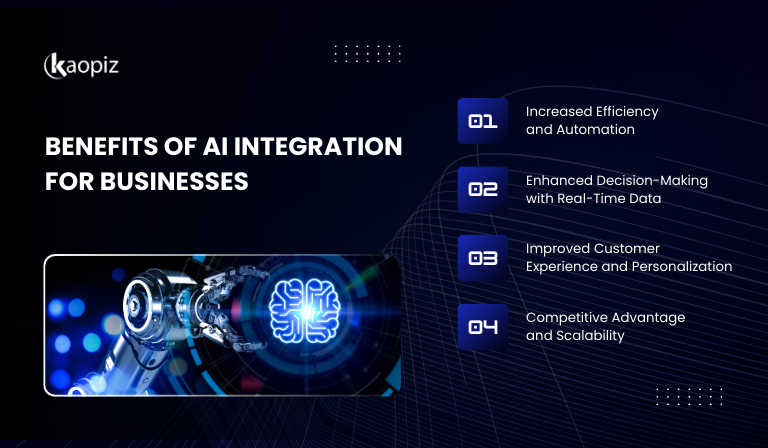
Here are the key reasons why AI integration matters for businesses:
Real-World Applications of AI Integration (with Case Studies)
This process is transforming industries across the board—here are real-world examples of how businesses are leveraging AI to drive innovation, efficiency, and growth.
Customer Service
In customer service, AI is enabling faster, smarter, and more personalized support across channels.
Key Applications in Customer Service:
Case study: Bank of America – AI-Powered Customer Support
Aspect | Details |
---|---|
Challenge | Manual handling of millions of inquiries led to long wait times and high costs |
AI Integration |
|
Results |
|
Manufacturing
AI integration transforms manufacturing operations by improving efficiency, accuracy, and reliability. From predictive maintenance to real-time quality control, manufacturers use AI to reduce downtime, cut costs, and increase output.
Key Applications in Manufacturing:
Case Study: Siemens – AI for Predictive Maintenance
Aspect | Details |
---|---|
Challenge | High downtime, labor shortages, and the need for safer, more efficient industrial operations. |
AI Integration |
|
Results |
|
Legal
AI is automating time-consuming tasks like contract review, legal research, and risk assessment. By integrating AI, legal teams can improve accuracy, reduce costs, and increase their capacity to handle growing workloads without expanding headcount.
Key Applications in Legal:
Case Study: Luminance – AI for Contract Review
Aspect | Details |
---|---|
Challenge | A lean legal team struggled to manage and review 50+ contracts daily, creating bottlenecks and delaying decision-making. |
AI Integration |
|
Results |
|
Healthcare
AI integration in healthcare is revolutionizing the way providers diagnose conditions, personalize treatments, and manage operations.
Key Applications in Healthcare:
Case Study: IBM Watson Health – AI in Diagnosis & Drug Discovery
Aspect | Details |
---|---|
Challenge | Physicians faced difficulty diagnosing complex diseases due to the overwhelming volume of patient data. |
AI Integration |
|
Results |
|
Education
AI integration in education is enhancing learning experiences, streamlining administrative processes, and enabling personalized instruction.
Key Applications in Education:
Case Study: Gradescope – AI Grading Tool for Educators
Aspect | Details |
---|---|
Challenge | Manual grading was time-consuming and delayed feedback for students. |
AI Integration | Implemented AI to group and score assignments, provide per-question analytics, and streamline feedback. |
Results |
|
Top Challenges of AI Integration
While AI integration in business offers transformative benefits, it also comes with a unique set of challenges. For organizations to unlock AI’s full potential, they must navigate technical, operational, and ethical obstacles that can impact success and scalability.
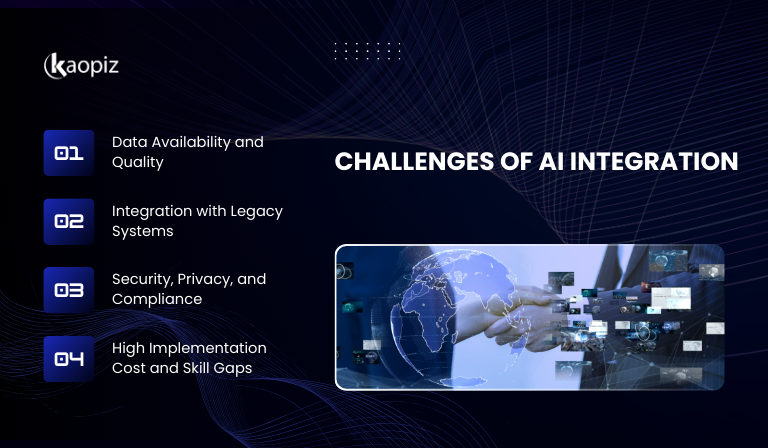
- Data Availability and Quality
AI systems require large volumes of high-quality, well-structured data. Many organizations struggle with fragmented, outdated, or biased data—making it difficult to train reliable models.
- Integration with Legacy Systems
Legacy infrastructure is often incompatible with modern AI tools. Bridging the gap between old and new systems can require significant time, customization, and technical expertise.
- Security, Privacy, and Compliance
AI systems handle sensitive information, raising concerns around data privacy, model transparency, and regulatory compliance (e.g., GDPR, HIPAA). Ensuring ethical and secure AI usage is critical.
- High Implementation Cost and Skill Gaps
Building and maintaining AI solutions can be costly. In addition, there’s a global shortage of AI specialists, making it difficult for many businesses to access the expertise required for successful integration.
AI Integration Strategies for Long-Term Success
Successfully integrating AI into your business is not a one-time deployment—it’s a long-term transformation that requires strategic alignment, cross-functional collaboration, and continuous improvement.
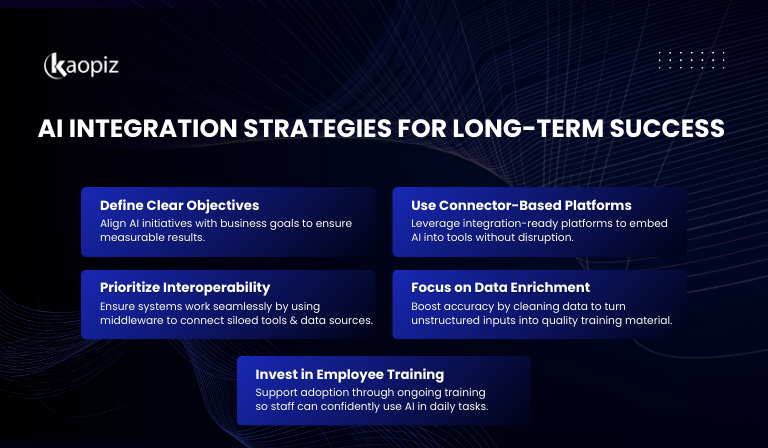
Below are key strategies to ensure AI delivers meaningful and sustainable impact:
The Future of AI Integration
AI integration is rapidly evolving from a differentiator to a business essential. As organizations embrace intelligent automation, personalization, and data-driven decision-making, AI will move deeper into core business operations. Here’s what the future holds:
- Edge AI Will Power Real-Time Decisions
AI models will increasingly run on local devices, reducing latency, enhancing privacy, and cutting cloud dependency. This is a game-changer for autonomous vehicles, IoT manufacturing, and real-time analytics.
- AI-Generated Code Will Accelerate Software Development
AI tools like GitHub Copilot and similar platforms will streamline coding, reduce development time, and lower the barrier to entry for software creation, empowering teams to build applications with minimal manual effort.
- Autonomous AI Agents Will Manage Complex Tasks
Businesses will deploy intelligent agents capable of independently executing operations, from inventory management to customer support, making real-time decisions without human input.
- Ethics and Governance Will Take Center Stage
With AI influencing everything from hiring to healthcare, transparency, bias mitigation, and regulatory compliance will become critical. Companies will need robust frameworks for responsible AI deployment.
How Kaopiz Helps Businesses with AI Integration
At Kaopiz, we help organizations turn AI from a buzzword into a business advantage. Whether you’re exploring automation, predictive analytics, or generative AI, our team provides end-to-end AI integration services tailored to your industry, infrastructure, and growth goals.
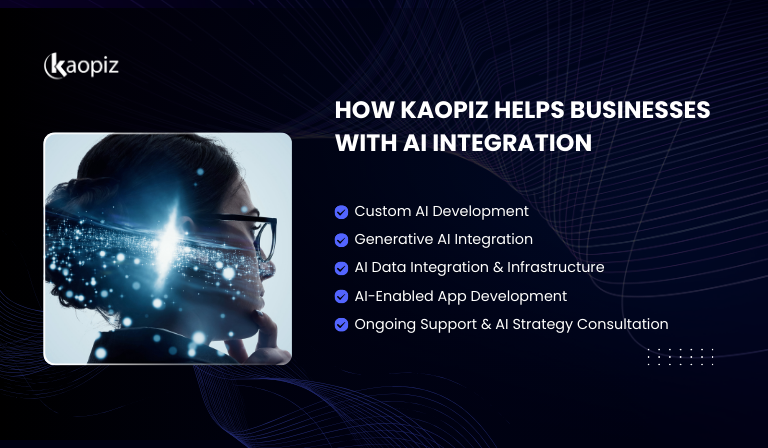
Our AI Integration Services Include:
If you are wondering how to integrate AI into an app or a system, our AI integration specialists provide the technical expertise and strategic insight to make integration smooth, secure, and impactful.
Conclusion
AI integration is no longer optional—it’s a strategic imperative for businesses looking to stay competitive, agile, and innovative in 2025 and beyond. From automating repetitive tasks to enhancing decision-making and delivering personalized experiences, AI has the power to transform every corner of an organization.
However, successful integration demands clear goals, clean data, the right technology partners, and a long-term vision. By investing in the right strategy and working with trusted software development companies like Kaopiz, businesses can unlock AI’s full potential and create scalable, intelligent systems that drive real results.
FAQs
Is It Necessary to Include AI in Development Projects?
While not always mandatory, integrating AI into development projects is increasingly essential for businesses looking to automate processes, enhance user experiences, and stay competitive. AI adds value through personalization, predictive insights, and intelligent automation, making products smarter and more scalable.
How Can AI Be Integrated into Product Infrastructure?
AI can be integrated through APIs, SDKs, or custom models embedded into your app or backend systems. This may involve using third-party AI platforms (e.g., OpenAI, AWS, Azure) or building your models for functions like recommendations, computer vision, or natural language processing. Successful integration also requires proper data pipelines, model deployment tools, and infrastructure support.
How Long Does It Take to Integrate AI for a Business?
The timeline depends on the complexity of the use case and the company’s readiness. A basic integration (e.g., chatbot or recommendation engine) can take 4–8 weeks, while enterprise-scale AI systems may require several months. Factors include data availability, existing infrastructure, model training, testing, and cross-functional coordination.
Trending Post
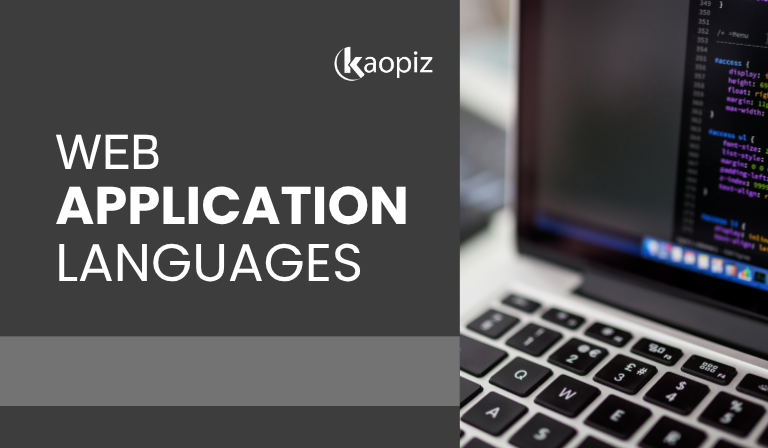
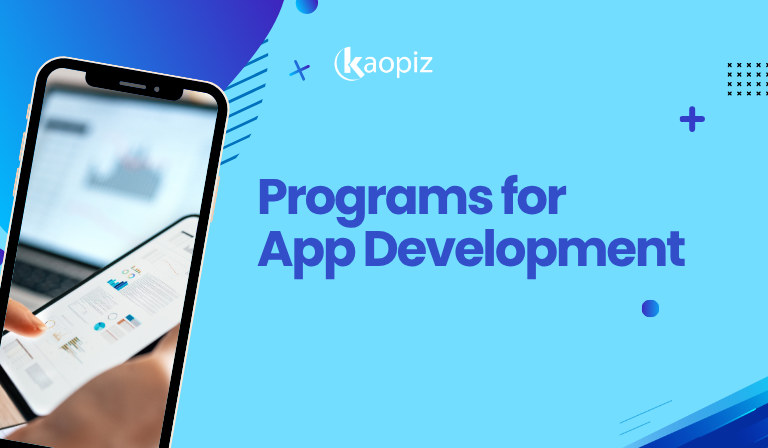
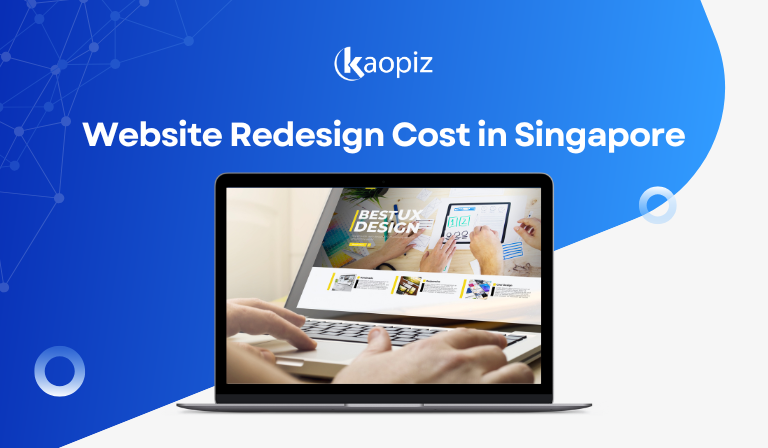
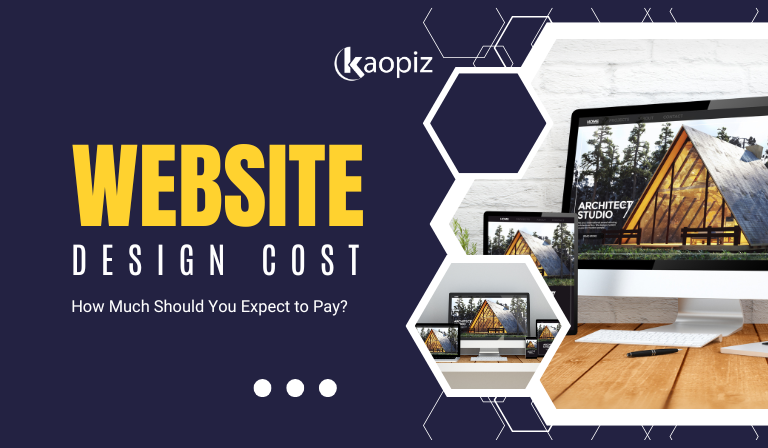